Statistical analysis has been used successfully in the business world for a long time. However, with the development of machine learning technologies and data-driven solutions, new avenues have opened up in this field. Modern predictive models offer businesses the ability to accurately predict user behavior and the likelihood of certain actions and events, making them a valuable technology in many areas, including marketing.
There are many prominent areas where predictive models are used. These include retail to increase product range and upsell, banking to assess customer solvency, and manufacturing to track equipment. Additionally, predictive models are used in marketing to determine the impact of advertising campaigns on businesses and users.
How do predictive models help?
Predictive models can support you in marketing and related fields. Let’s look at a few examples:
- Determine the probability of an event. When you have more data on the various factors that can influence an event, you can more accurately predict the outcome.
- Predict how a specific audience segment will perceive your advertising campaign.
- Determine the optimal cost for in-app purchases.
- Choose the best optimization options, KPIs and budget for your marketing campaign.
- Find customers with the highest LTV or another key metric.
Let’s look at the last scenario in more detail.
What is LTV and why is it important?
Customer lifetime value (LTV) is a critical metric that helps you understand how much revenue the average user of your app generates. This metric is critical when calculating your return on investment in user acquisition, user engagement, and retention.
The marketing industry has begun to pay more attention to LTV due to rising customer attraction costs. Ultimately, the success of their efforts depends on the LTV of the majority of users. Attracting a user often costs more than the initial purchase, so companies compare cost of acquisition (CPA) to LTV. The higher the LTV, the more likely the promotion will deliver results.
Understanding how much revenue a potential user will bring not only on the first transaction, but throughout the entire period of activity, will allow you to quickly develop your marketing strategy and allocate your budget wisely.
How does the LTV prediction model help user acquisition app campaigns?
Based on how users behave on their first day in an app, a machine learning model can predict how much time they will spend in similar apps and how much revenue app owners will generate.
This model is trained with anonymized data from similar apps and revenues or user retention data sent to the advertising platform. The advertising platform also adjusts bids in real-time and attracts users with the promise of a higher LTV for your app. You can review some examples of this entire process below.
So how do standard user acquisition algorithms based on app installation prediction work? Ads are shown to users who are most likely to install the app. For example:
You can see how the LTV-driven user acquisition algorithm works: The system takes into account the potential LTV of users and finds the highest quality audience:
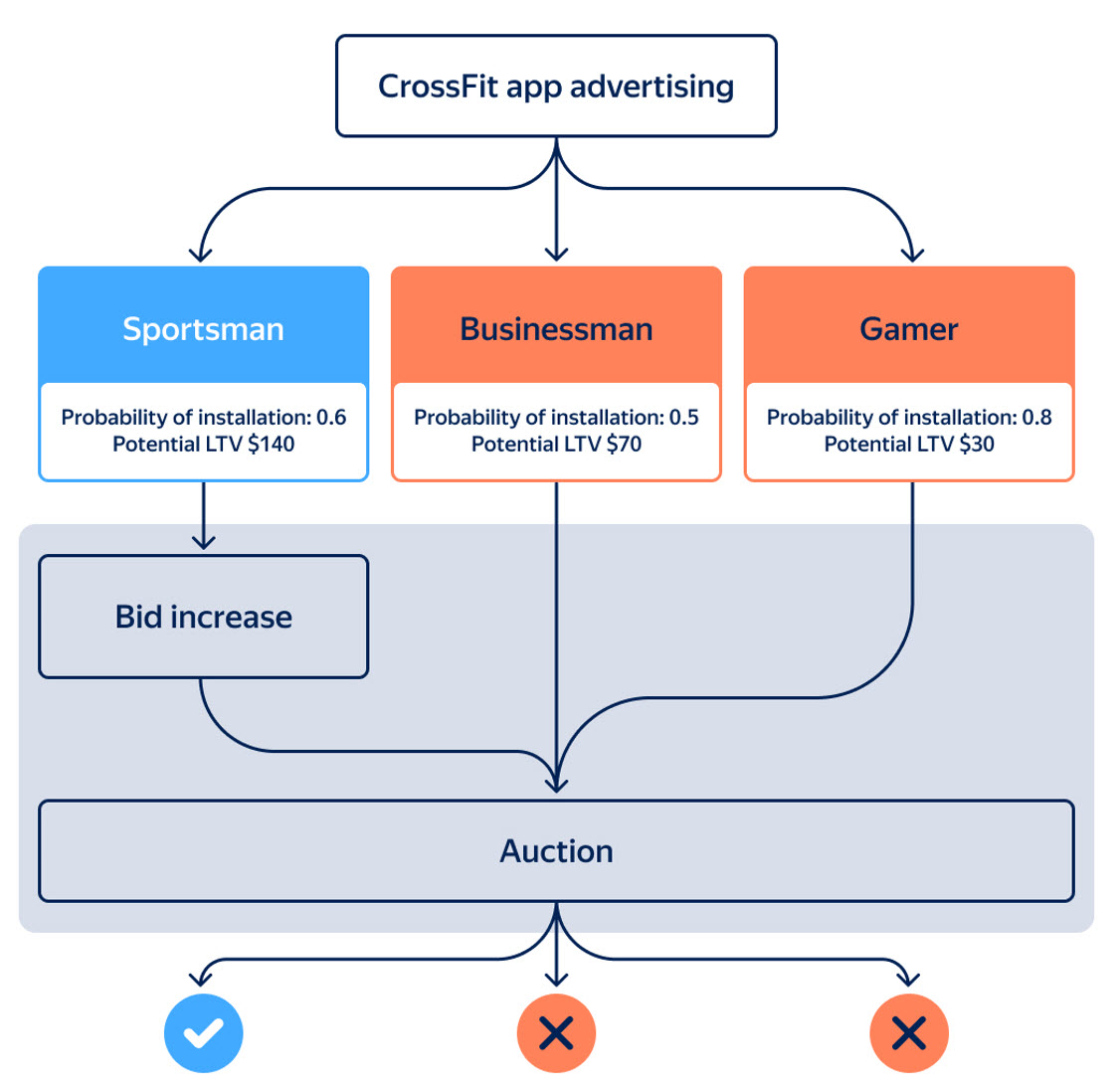
How was the LTV estimation algorithm developed?
On the other hand, businesses may not always have enough data or analysts to calculate LTV. To overcome this challenge, we automated this process and implemented various predictive models in Yandex Direct, Yandex’s own platform for advertisers. This innovation allows advertisers promoting mobile apps to see more post-install conversions and generate more revenue, especially in pay-per-install campaigns.
AppMetrica, Yandex Ads’ mobile analytics platform, has a score unit that estimates LTV for app users. We used this score to train our models and included the probability of targeted activities post-installation in our predictions. This score will be the key factor for selecting ads in automated strategies.
The new approach increases overall revenue by significantly increasing the number of targeted activities after installs. The effect on pay-per-install campaigns is particularly striking: while in the testing phase we observed a profit increase of up to 12% from acquired users.
What do you think about this issue? Share your opinions in the comments!